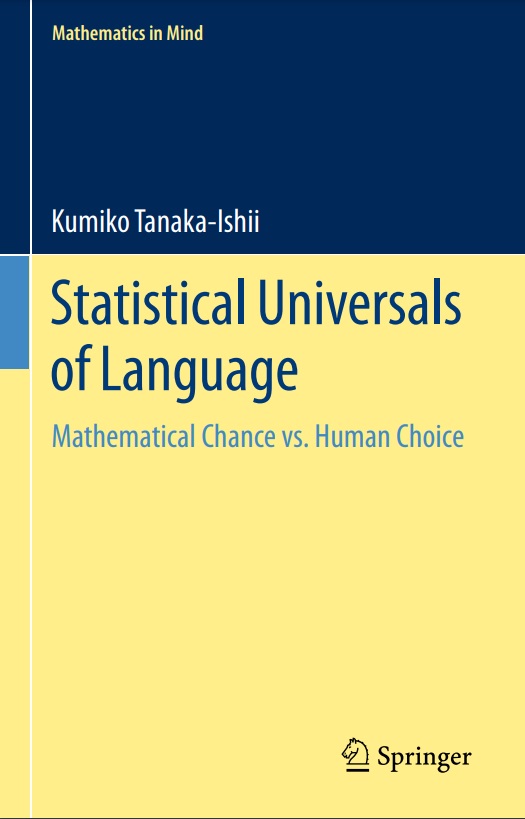
Title
Mathematics in Mind Statistical Universals of Language Mathematical Chance vs. Human Choice
Size
236 pages, hardcover
Language
English
Released
2021
ISBN
978-3-030-59376-6
Published by
Springer Cham
Book Info
See Book Availability at Library
Japanese Page
This book proposes a conjecture of natural language as a complex system.
Natural language has multiple global macroscopic properties, including the well-known Zipf’s law. It takes the form of power laws that represent self-similarity properties. The nature of these properties has mainly been studied within complex system science, a field that is outside both linguistics, involving traditional scientific studies of language, and natural language processing, which is an engineering research field. The frontier of complex system studies on natural language has advanced through publications that are distributed across various journals. Since the frontier is difficult to see, this book attempts to explain it in a consistent and unified manner.
In summary, this book discusses the following themes:
1. What are the macroscopic properties of language? (Parts 2 and 3)
2. How are the macroscopic and microscopic properties related? (Part 4)
3. What is their significance in engineering? (Part 5)
Regarding theme 1, laws such as Heaps’ law are known to be derived from Zipf’s law. This book provides an overview of the relations among them. Thereafter, it considers Zipf’s law as a limit theorem. In other words, it proposes that language is established on such an inevitable statistical property. Furthermore, in addition to Zipf’s law on the distribution of words, the macroscopic nature of language lies in the property of word sequences. The author’s studies along that line also yield multiple power laws, which characterize the self-similarity that underlies the fluctuation of context.
Such macroscopic properties influence the microscopic formation of language elements, grammatical structure, and semantic properties. Theme 2 addresses their possible relations, indicating how microscopic linguistic properties could be derived from macroscopic properties.
Theme 3 considers the significance of the macroscopic properties in engineering. Currently, natural language documents are processed from sequence to sequence through deep learning. The macroscopic perspective on language presented in this book could provide a formal basis for such language processing. One fundamental component of natural language processing is the language model. Macroscopic properties also enable the analysis of previous language models. Indeed, one explanation for the recent leap in AI via deep learning is that the models have begun to satisfy the macroscopic properties presented in this book.
Lastly, this book is also a sequel to the author’s previous book entitled, Semiotics of Programming, which argued how a sign is inherently recursive. Accordingly, the whole system of signs is destined to be reflexive, resulting in a phenomenon referred to as “structure” in structuralism. It then answers, what kind of property is “structure?” This book attempts to factor this structure from a viewpoint of self-similarity, as shown by various power laws that it carefully explains.
(Written by TANAKA-ISHII Kumiko, Professor, Research Center for Advanced Science and Technology / 2022)
Table of Contents
1 Introduction
1.1 Aims
1.2 Structure of This Book
1.3 Position of This Book
1.4 Prospectus
2 Universals
2.1 Language Universals
2.2 Layers of Universals
2.3 Universal, Stylized Hypothesis, and Law
3 Language as a Complex System
3.1 Sequence and Corpus
3.2 Power Functions
3.3 Self-Free Property:Statistical Self-Smilarity
3.4 Complex Systems
3.5 Two Basic Random Processes
Part II Property of Population
4 Relation Between Rank and Frequency
4.1 Zipf’s Law
4.2 Scale-Free Property and Hapax Legomena
4.3 Monkey Text
4.4 Power Law of n-grams
4.5 Relative Rank-Frequency Distribution
5 Bias in Rank-Frequency Relation
5.1 Literary Texts
5.2 Speech, Music, Programs, and More
5.3 Deviation from Power Law
5.4 Nature of Deviation
6 Related Statistical Universals
6.1 Density Function
6.2 Vocabulary Growth
Part III Property of Sequences
7 Returns
7.1 Word Returns
7.2 Distribution of Return Interval Lengths
7.3 Exceedance Probability
7.4 Bias Underlying Return Intervals
7.5 Rare Words as a Set
7.6 Behavior of Rare Words
8 Long-Range Correlation
8.1 Long-Range Correlation Analysis
8.2 Mutual Information
8.3 Autocorrelation Function
8.4 Correlation of Word Intervals
8.5 Nonstationarity of Language
8.6 Weak Long-Range Correlation
9 Fluctuation
9.1 Fluctuation Analysis
9.2 Taylor Analysis
9.3 Differences Between the Two Fluctuation Analyses
9.4 Dimensions of Linguistic Fluctuation
9.5 Relations Among Methods
10 Complexity
10.1 Complexity of Sequence
10.2 Entropy Ratep
10.3 Hilberg’s Ansatz
10.4 Computing Entropy Rate of Human Language
10.5 Reconsidering the Question of Entropy Rate
Part IV Relation to Linguistic Elements and Structure
11 Articulation of Elements
11.1 Harris’ Hypothesis
11.2 Information-Theoretic Reformulation
11.3 Accuracy of Articulation by Harris’ Scheme
12 Word Meaning and Value
12.1 Meaning as Use and Distibutional Senmantics
12.2 Weber-Fechner Law
12.3 Word Frequency and Familiarity
12.4 Vector Representation of Words
12.5 Compositionality of Meaning
12.6 Statistical Universals and Meaning
13 Size and Frequency
13.1 Zipf Abbreviation of Words
13.2 Compound Length and Frequency
14 Grammatical Structure and Long Memory
14.1 Simple Grammatical Framework
14.2 Phrase Structure Grammar
14.3 Long-Range Dependence in Sentences
14.4 Grammatical Structure and Long-Range Correlation
14.5 Nature of Long Memory Underlying Language
Part V Mathematical Models
15 Theories Behind Zipf’s Law
15.1 Communication Optimization
15.2 A Limit Theorem
15.3 Signification of Statistical Universals
16 Mathematical Generative Models
16.1 Criteria for Statistical Universals
16.2 Independent and Identically Distributed Sequences
16.3 Simon Model and Variants
16.4 Random Walk Models
17 Language Models
17.1 Language Models and Statistical Universals
17.2 Building Language Models
17.3 N-Gram Models
17.4 Grammatical Models
17.5 Neural Models
17.6 Future Directions for Generative Models
Part VI Ending Remarks
18 Conclusion
19 Acknowledgments
PartVII Appendix
20 Glossary and Notations
20.1 Glossary
20.2 Mathematical Notation
20.3 Other Conventions
21 Mathematical Details
21.1 Fitting Functions
21.2 Proof that Monkey Typing Follows a Power Law
21.3 Relation Between η and ζ
21.4 Relation Between η and ξ
21.5 Proof That Interval Lengths of I.I.D. Process Follow Exponential Distribution
21.6 Proof of α = 0.5 and ν = 1.0 for I.I.D. Process
21.7 Summary of Shannon’s Method to Estimate Entropy Rate
21.8 Relation of h, Perplexity, and Cross Entropy
21.9 Type Counts, Shannon Entropy, and Yule’s K, via Generalized Entropy
21.10 Upper Bound of Compositional Distance
21.11 Rough Summary of Mandelbrot’s Communication Optimization Rationale to Deduce a Power Law
21.12 Rough Definition of Central Limit Theorem
21.13 Definition of Simon Model
22 Data
22.1 Literary Texts
22.2 Large Corpora
22.3 Other Kinds of Data Related to Language
22.4 Corpora for Scripts
References
Index
Related Info
The Mainichi Publishing Culture Award (Mainichi newspaper Nov. 2021)
Review:
Reviewed by FIRDOUS AHMAD MALA
The book provides excellent food for thought. It makes the reader think of untrodden paths. It questions a big binary, the binary of language being completely different from natural and social sciences (Rising Kashmir Jan 25, 2022)
https://www.risingkashmir.com/Statistical-Universals-of-Language-99573
Japanese Edition:
“Gengo to Fractal” published by the University of Tokyo Press, in 2021
http://www.utp.or.jp/book/b559376.html