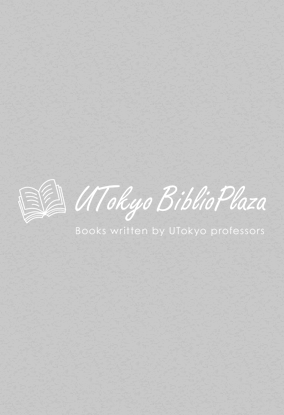
Title
SIAM Journal on Scientific Computing (Volume 45, Issue 2) Dynamic finite-budget allocation of stratified sampling with adaptive variance reduction by strata
Language
English
Released
2023
ISSN
1064-8275
Published by
Society for Industrial and Applied Mathematics
Book Info
See Book Availability at Library
Japanese Page
Stochastic models have continued to grow infinitely larger and more complex for practical use, and thus now require extremely heavy computing to gain insights from such stochastic models. Conversely, the steady advancement of computing power has opened the door for putting large-scale and complex stochastic models into practice as well. This chicken-and-egg phenomenon is prominent not only in the cutting-edge technology fields of data science and AI, but has also been prevalent in the conventional fields of humanities and social sciences for a very long time, such as econometrics, business administration, and even sociology, to name just a few. In particular, results and implications derived from such extremely heavy numerical experiments have been truly indispensable for investment strategies, management decisions, and social analysis.
Ideally, one would like to know in advance how much computing time would be required for obtaining decent outputs and how close those would be to the unknown true value. Computing without a clue could otherwise be reckless, resulting in a waste of precious time and computing resources, including electricity. Clearly, this would be more and fatally pronounced in large-scale computing at the implementation level.
This work provides a systematic analysis of large-scale computing with various methods employed in a general format alongside, specifically, variance reduction methods such as importance sampling, control variates, and stratified sampling, through convergence and error analyses. Equally important is that this work extends the orthodox scope further to address important practical issues, such as the optimal execution of computing resources and optimal stopping of iterative computation, each of which comes to light upon implementation without an exception. The author hopes that the gained prior knowledge on heavy computing of large-scale and complex stochastic models will prove to be of great assistance in developing, for instance, investment strategies and business decisions more effectively.
(Written by KAWAI Reiichiro, Professor, Graduate School of Arts and Sciences / 2023)