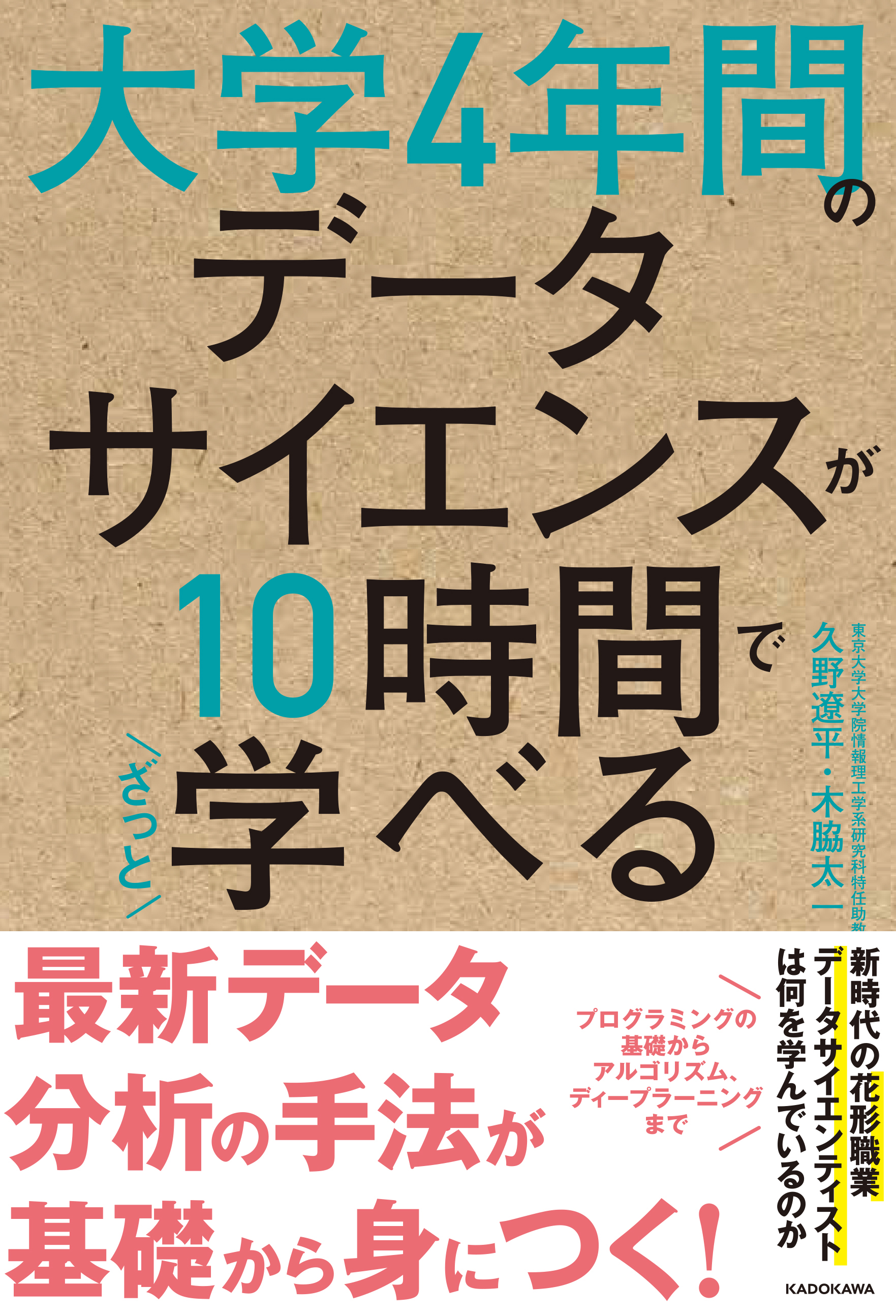
Title
Daigaku Yo-nenkan no Data Science ga 10-jikan de Zatto Manaberu (Learn 4 Years’ Worth of College Data Science in Just 10 Hours!)
Size
256 pages, 127x188mm
Language
Japanese
Released
March 29, 2018
ISBN
9784046022110
Published by
KADOKAWA
Book Info
See Book Availability at Library
Daigaku Yo-nenkan no Data Science ga 10-jikan de Zatto Manaberu
Japanese Page
Data science is a fast-moving field. When I revisited this volume so that I could write this commentary, I noticed several sections whose contents I would change were I to write it again. This realization confirms that there have been many breakthroughs over the last 5 years since I first started writing this book.
What particularly caught my attention was the deep learning section. Nowadays, it is impossible to delve into this topic without also explaining the progress of natural language processing and image processing technology triggered by transformer technology. The learning theory of deep learning has also made great progress, which renders it impossible to imagine the topic of benign overfitting based on the details explained in this volume. In recent years, there has been a very active movement to publish pre-trained deep-learning models trained on a large-scale dataset. Pre-training models can be downloaded with a single click and easily used even by beginners. In light of these developments, even beginners may need to rethink the scope of the knowledge they want to gain, even if only roughly.
Furthermore, changes have been occurring not only in cutting-edge machine learning but also in the way in which data scientists work. As the author of this volume, I have also been involved in practical data science education for a long time. In the early days, I remember that one could make a living simply by tuning the machine learning models properly. However, with the advent of automated machine learning technology, more and more of those tasks are being performed by machines. As such, people with knowledge of domains – which are the source of data – can sometimes build models that are faster and more accurate than those solely knowledgeable in machine learning techniques. Undoubtedly, without a good understanding of statistics, econometrics, and machine learning, one will end up writing software with incorrect interpretations or low universality. In the real world of decision making, however, an 80-point answer reached in a week using automated machine learning techniques by someone who does not have a perfect understanding of data science but is familiar with the underlying events and business issues behind the problem being solved may sometimes be preferred over a 95-point answer given in 6 months by a professional data scientist. This is because some people consider rapid decision making to be more important. Thankfully, this current accuracy gap is gradually closing, which means that it may not be long before we will be separately teaching "data science that carefully understands theory from scratch" and “data science that leverages domain knowledge with machine assistance.”
Such modern innovations and realities are, unfortunately, not documented in this current volume. Then, does it mean that this book has no relevance in the present time? I do not think so. Regardless of the era, there is always some kind of fundamental knowledge that flows throughout. Keeping in mind that this is a changing field, it may be beneficial to read this book while questioning what this true knowledge is. Indeed, what makes the field of data science exciting is the very fact that it is constantly evolving.
(Written by HISANO Ryohei, Lecturer, Graduate School of Information Science and Technology / 2023)