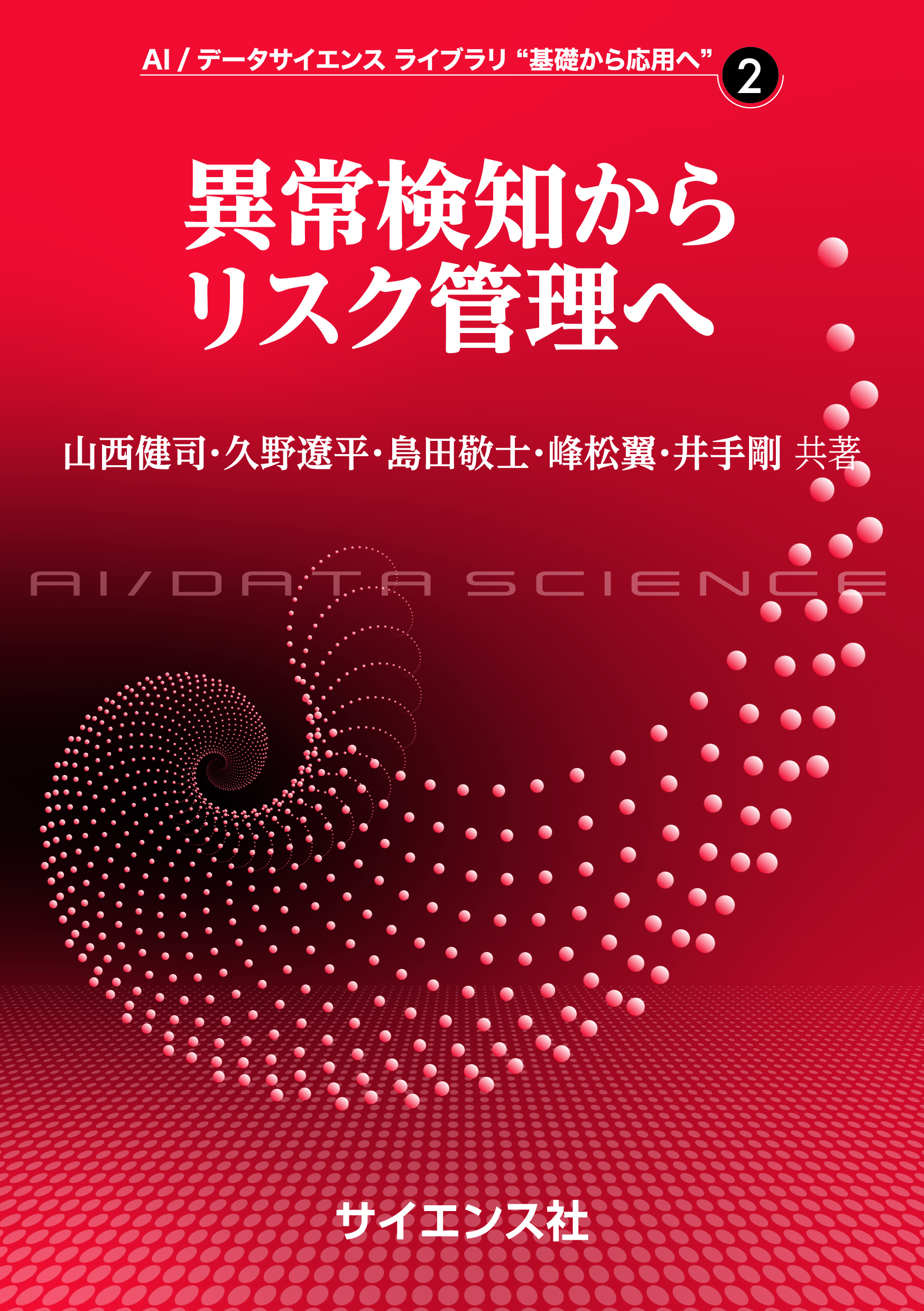
Title
AI / Data Science Series — From Theory to Practice 2 Ijo-kenchi kara Risk-kanri e (From Anomaly Detection to Risk Management)
Size
208 pages, A5 format, softcover
Language
Japanese
Released
August 25, 2022
ISBN
978-4-7819-1546-3
Published by
SAIENSU-SHA Co.,Ltd,
Book Info
See Book Availability at Library
Japanese Page
This book introduces anomaly detection, which is one of most important technologies in data science. This technology aims to efficiently and effectively detect anomalies such as outliers or changes from a large amount of data. Anomaly detection has been applied to a wide range of areas including marketing, security, medicine, finance. This book shows a fundamental theory of anomaly detection with its applications to risk management, specifically focusing on economics, education, and security. A feature of this book is that it is written with an emphasis on building a bridge from basic theory to application.
Chapter 1 gives a survey of fundamental theory of anomaly detection. In it, the technologies of anomaly detection are categorized into two; outlier detection and change detection. The former aims to detect data points that are largely deviated from other points, while the latter aims at detecting significant changes of data sources over time. Both of them are based on machine learning of regularities for data sources. A feature of this book is that it explains machine learning from a perspective of “information-theoretic learning theory.” This theory gives a unifying view of the evaluation of machine learning model in terms of how well the data sequence is compressed through that model.
Chapter 2 shows an application of change detection to time series in economics. For example, when we apply change detection to time series of stock prices, the detected change points may correspond to serious economic events. This chapter shows that we possibly identify such events through change detection to financial data. For another example, we may construct a network by letting a node be a stock owner or stock itself and an edge be a stock ownership relation. This chapter shows that if we apply change detection to a stream of such networks, we are able to identify macroscopic changes of corporate governance.
Chapter 3 shows applications of anomaly detection to educational data mining. Nowadays, a huge amount of data about learning activities are being accumulated due to the development of digital learning environments. This chapter shows examples of applications of anomaly detection to such learning activity data. For example, when we apply change detection to click streams obtained in learning activities, it is possible to determine whether the effectiveness of teaching materials and classes has improved. In addition, by detecting outliers in the behavior of viewing digital learning materials, it is possible to identify learners whose behavior in viewing learning materials differs from that of the majority of learners. This allows for fine-grained learning support.
Chapter 4 introduces machine learning in a decentralized environment as a form of risk management. Considering a learning problem with three constraints; democracy, diversity, and privacy, decentralized learning can be reduced to the problem of secret aggregation. This chapter introduces several specific methods of secret aggregation.
Throughout this book, readers can learn not only a solid theory of anomaly detection but also a variety of its applications through plenty of examples.
(Written by YAMANISHI Kenji, Professor, Graduate School of Information Science and Technology / 2023)