A soft touch for robotic hardware Combined muscles and sensors made from soft materials allow for flexible robots Research news
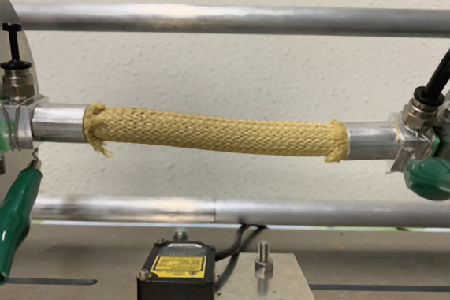
Robots can be made from soft materials, but the flexibility of such robots is limited by the inclusion of rigid sensors necessary for their control. Researchers created embedded sensors, to replace rigid sensors, that offer the same functionality but afford the robot greater flexibility. Soft robots can be more adaptable and resilient than more traditional rigid designs. The team used cutting-edge machine learning techniques to create their design.
Automation is an increasingly important subject, and core to this concept are the often paired fields of robotics and machine learning. The relationship between machine learning and robotics is not just limited to the behavioral control of robots, but is also important for their design and core functions. A robot which operates in the real world needs to understand its environment and itself in order to navigate and perform tasks.
If the world was entirely predictable, then a robot would be fine moving around without the need to learn anything new about its environment. But reality is unpredictable and ever changing, so machine learning helps robots adapt to unfamiliar situations. Although this is theoretically true for all robots, it is especially important for soft-bodied robots as the physical properties of these are intrinsically less predictable than their rigid counterparts.
“Take for example a robot with pneumatic artificial muscles (PAM), rubber and fiber-based fluid-driven systems which expand and contract to move,” said Associate Professor Kohei Nakajima from the Graduate School of Information Science and Technology. “PAMs inherently suffer random mechanical noise and hysteresis, which is essentially material stress over time. Accurate laser-based monitors help maintain control through feedback, but these rigid sensors restrict a robot's movement, so we came up with something new.”
Nakajima and his team thought if they could model a PAM in real time, then they could maintain good control of it. However, given the ever-changing nature of PAMs, this is not realistic with traditional methods of mechanical modeling. So the team turned to a powerful and established machine learning technique called reservoir computing. This is where information about a system, in this case the PAM, is fed into a special artificial neural network in real time, so the model is ever changing and thus adapts to the environment.
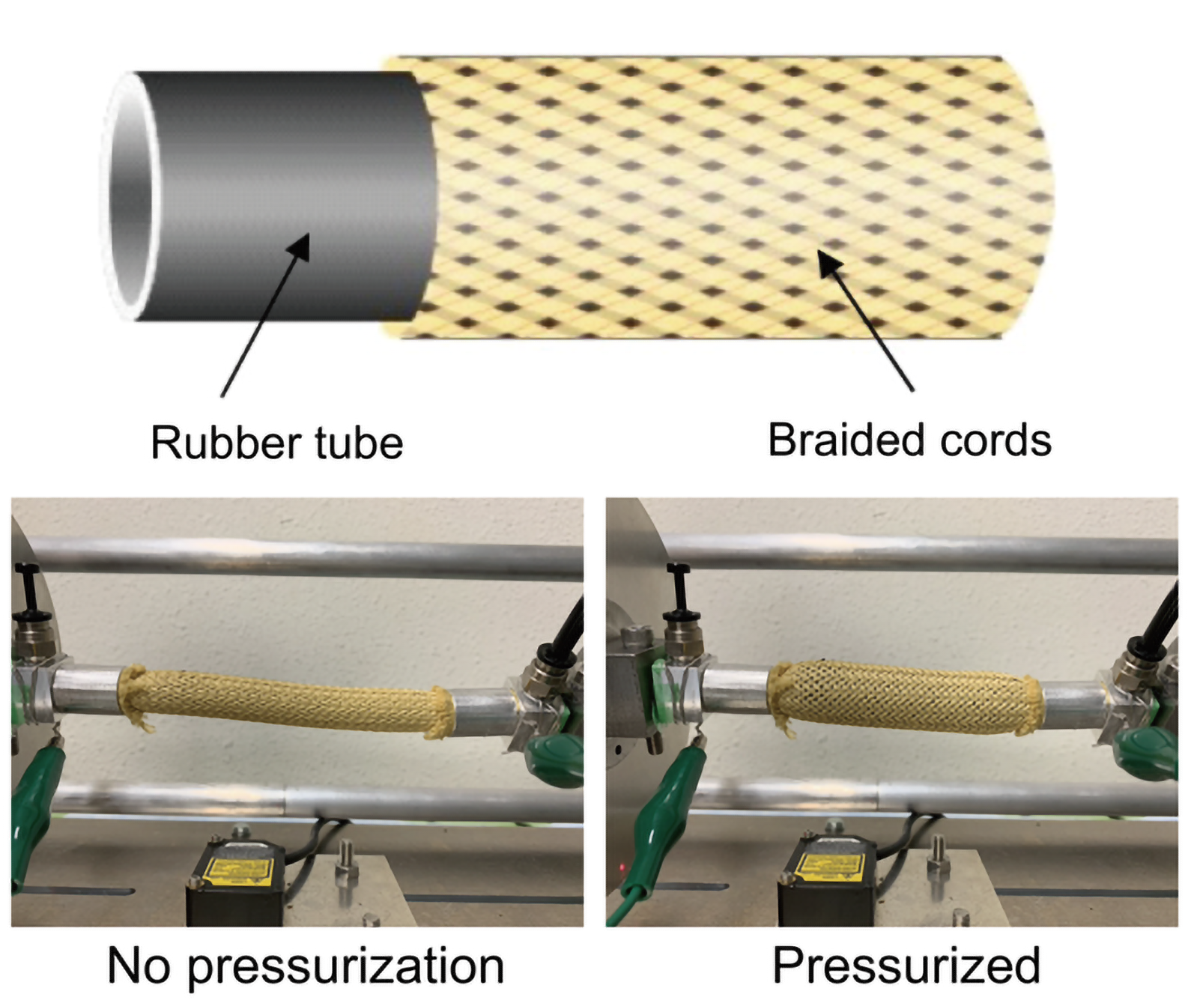
Pneumatic artificial muscle. A soft tube expands and contracts to create movement. © 2020 Nakajima et al.
“We found the electrical resistance of PAM material changes depending on its shape during a contraction. So we pass this data to the network so it can accurately report on the state of the PAM,” said Nakajima. “Ordinary rubber is an insulator, so we incorporated carbon into our material to more easily read its varying resistance. We found the system emulated the existing laser-displacement sensor with equally high accuracy in a range of test conditions.”
Thanks to this method, a new generation of soft robotic technology may be possible. This could include robots that work with humans, for example wearable rehabilitation devices or biomedical robots, as the extra soft touch means interactions with them are gentle and safe.
“Our study suggests reservoir computing could be used in applications besides robotics. Remote-sensing applications, which need real-time information processed in a decentralized manner, could greatly benefit,” said Nakajima. “And other researchers who study neuromorphic computing — intelligent computer systems — might also be able to incorporate our ideas into their own work to improve the performance of their systems.”
Papers
Ryo Sakurai, Mitsuhiro Nishida, Hideyuki Sakurai, Yasumichi Wakao, Nozomi Akashi, Yasuo Kuniyoshi, Yuna Minami, Kohei Nakajima, "Emulating a sensor using soft material dynamics: A reservoir computing approach to pneumatic artificial muscle," Proceedings of the IEEE International Conference on Soft Robotics (RoboSoft) 2020: May 15, 2020
Related links
- Next Generation Artificial Intelligence Research Center
- Department of Creative Informatics
- Graduate School of Information Science and Technology
- RoboSoft 2020 - IEEE conference on soft robotics