Seeing is deceiving A new way to train deepfake detection algorithms improves their success Research news
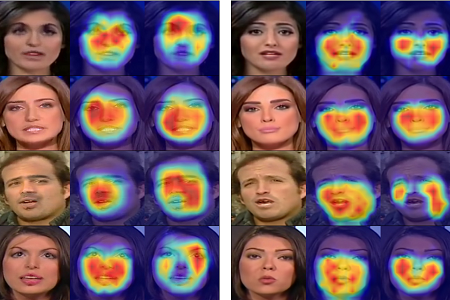
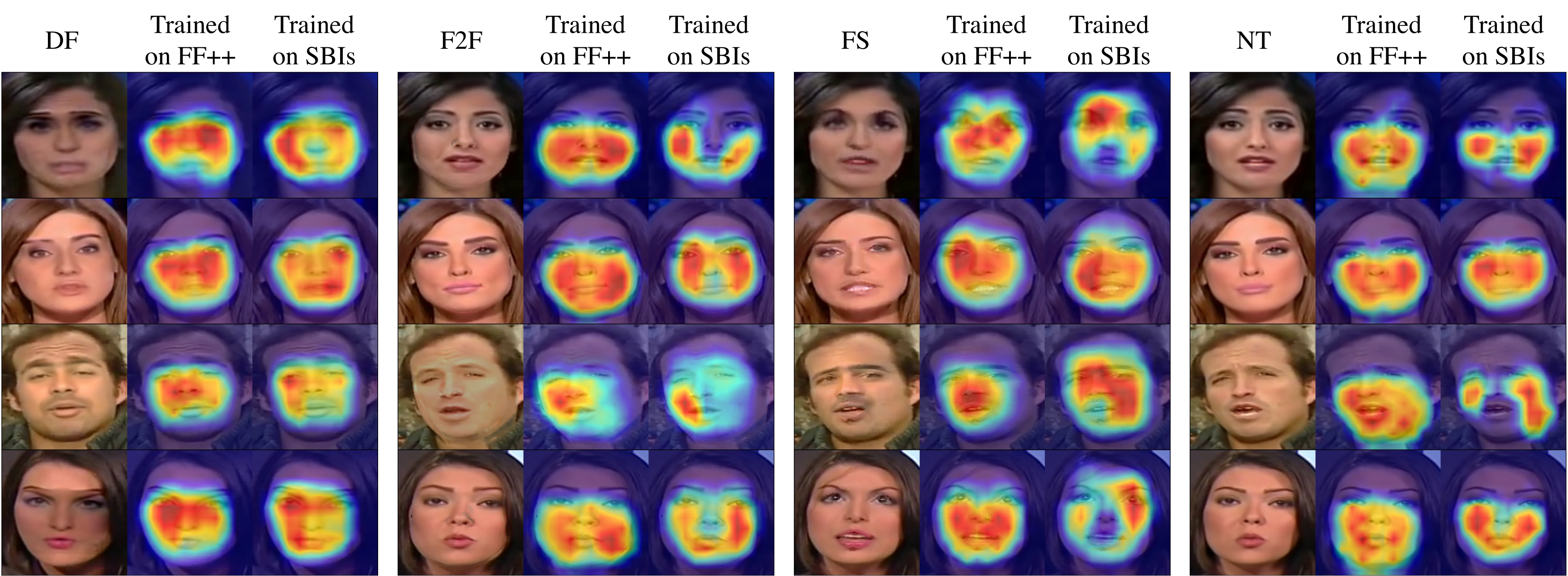
Spot the difference. An example of some deepfake photos were made using different manipulation methods (DF, F2F, FS and NT). A deepfake detector was then trained using an established data set of sample deepfakes (FF++), while a duplicate detector was trained using the researchers’ self-blended images (SBIs). The two detectors were given the above deepfake photos. The columns of false color images show the difference between training using existing data sets and training using SBIs. ©2022 Yamasaki and Shiohara
Deepfakes are images and videos which combine mixed source material to produce a synthetic result. Their use ranges from trivial to malicious, so methods to detect them are sought after, with the latest techniques often based on networks trained using pairs of original and synthesized images. A new method defies this convention by training algorithms using novel synthesized images created in a unique way. Known as self-blended images, these novel training data can demonstrably improve algorithms designed to spot deepfake images and video.
Seeing is believing, so they say. However, since the advent of recorded visual media, there have always been those who seek to deceive. Things range from the trivial, such as fake movies of UFOs, to far more serious matters such as the erasure of political figures from official photographs. Deepfakes are just the latest in a long line of manipulation techniques, and their ability to pass as convincing realities is far outpacing the progress of tools to spot them.
Associate Professor Toshihiko Yamasaki and graduate student Kaede Shiohara from the Computer Vision and Media Lab at the University of Tokyo explore vulnerabilities related to artificial intelligence, amongst other things. The issue of deepfakes caught their interest and they decided to investigate ways to improve detection of the synthetic content.
“There are many different methods to detect deepfakes, and also various sets of training data which can be used to develop new ones,” said Yamasaki. “The problem is the existing detection methods tend to perform well within the bounds of a training set, but less well across multiple data sets or, more crucially, when pit against state-of-the-art real world examples. We felt the way to improve successful detections might be to rethink the way in which training data are used. This led to us developing what we call self-blended images (otherwise known as SBIs).”
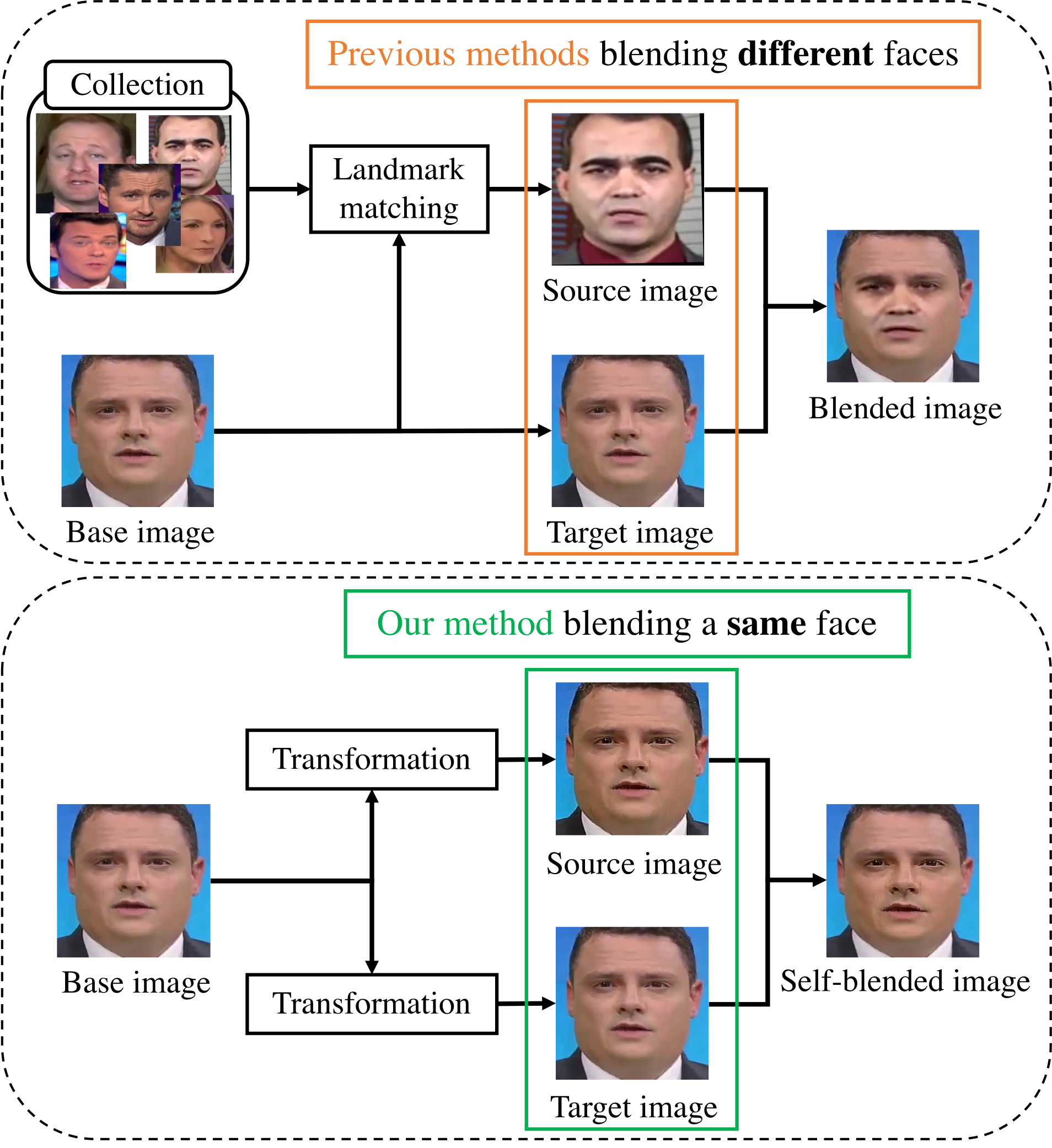
Blending images. The upper diagram shows the typical process of creating deepfakes for training data. The lower diagram shows the team’s way of making improved training data. ©2022 Yamasaki and Shiohara
Typical training data for deepfake detection consist of pairs of images, comprising an unmanipulated source image and a counterpart faked image — for example, where somebody’s face or entire body has been replaced with someone else’s. Training with this kind of data limited detection to certain kinds of visual corruption, or artifacts, resulting from manipulation, but missed others. So they experimented with training sets comprising synthesized images. This way, they could control the kinds of artifacts the training images contained, which could in turn better train detection algorithms to find such artifacts.
“Essentially, we took clean source images of people from established data sets and introduced different subtle artifacts resulting from, for example, resizing or reshaping the image,” said Yamasaki. “Then we blended that image with the original unaltered source. The process of blending these images would also depend on characteristics of the source image — basically a mask would be made so that only certain parts of the manipulated image would make it to the blended output. Many SBIs were compiled into our modified data set, which we then used to train detectors.”
The team found the modified data sets improved accurate detection rates by around 5-12%, depending on the original data set they were compared to. These might not sound like huge improvements, but it could make the difference between someone with malicious intent succeeding or failing to influence their target audience in some way.
“Naturally, we wish to improve upon this idea. At present, it works best on still images, but videos can have temporal artifacts we cannot yet detect. Also, deepfakes are usually only partially synthesized. We might also explore ways to detect entirely synthetic images, too,” said Yamasaki. “However, I envisage in the near future this kind of research might work its way onto social media platforms and other service providers so that they can better flag potentially manipulated images with some kind of warning.”
Papers
Kaede Shiohara and Toshihiko Yamasaki, "Detecting Deepfakes with Self-Blended Images," Proceedings of the IEEE/CVF Conference on Computer Vision and Pattern Recognition (CVPR), 2022: June 24, 2022
Link (Publication)