Quantum research with neural networks A novel way to simulate physical systems could accelerate physics research
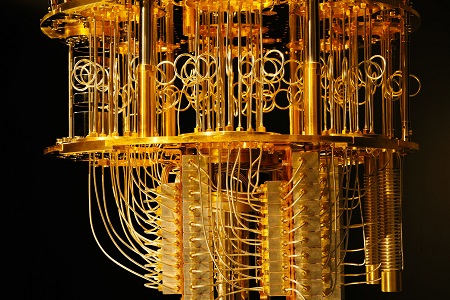
For the first time, researchers have applied a fundamental tool of machine learning to a difficult class of physical simulation. A specially developed neural network simplifies some of the complexity inherent in simulations of quantum mechanical systems. This research shows it is now possible to apply neural networks to a variety of highly complex physical simulations. One area this could be particularly useful is in the development of quantum computers.
You might want to buckle up for this; we’re going for a ride.
Quantum physics is the study of reality at small scales. It’s crucially important to everyday life as much technology relies on it, from smartphones to medical diagnosis equipment and more. Some quantum mechanical systems exhibit useful properties when in isolation, but lose these properties when there is some kind of interaction with their environment. Researchers believe if they study this process in detail, they may be able to create more robust quantum systems.
“This is the first time anyone has used neural networks to simulate a quantum system in a realistic setting, where it exchanges energy with its external environment,” said Nobuyuki Yoshioka, a doctoral student from the Institute for Physics of Intelligence. “The fragility of these systems makes them difficult to study in reality so a simulation is essential. Researchers looking to improve upon or create new quantum devices could make use of this.”
Although quantum systems have been studied in great detail using computers, the systems are generally the isolated kind. The problem is, when an isolated quantum system then interacts with the surrounding environment, the mathematics needed to describe the system becomes increasingly complex. So much so that even the world's largest supercomputers are hard pushed to run these simulations. Researchers needed a way to reduce this complexity.
This is where neural networks come in.
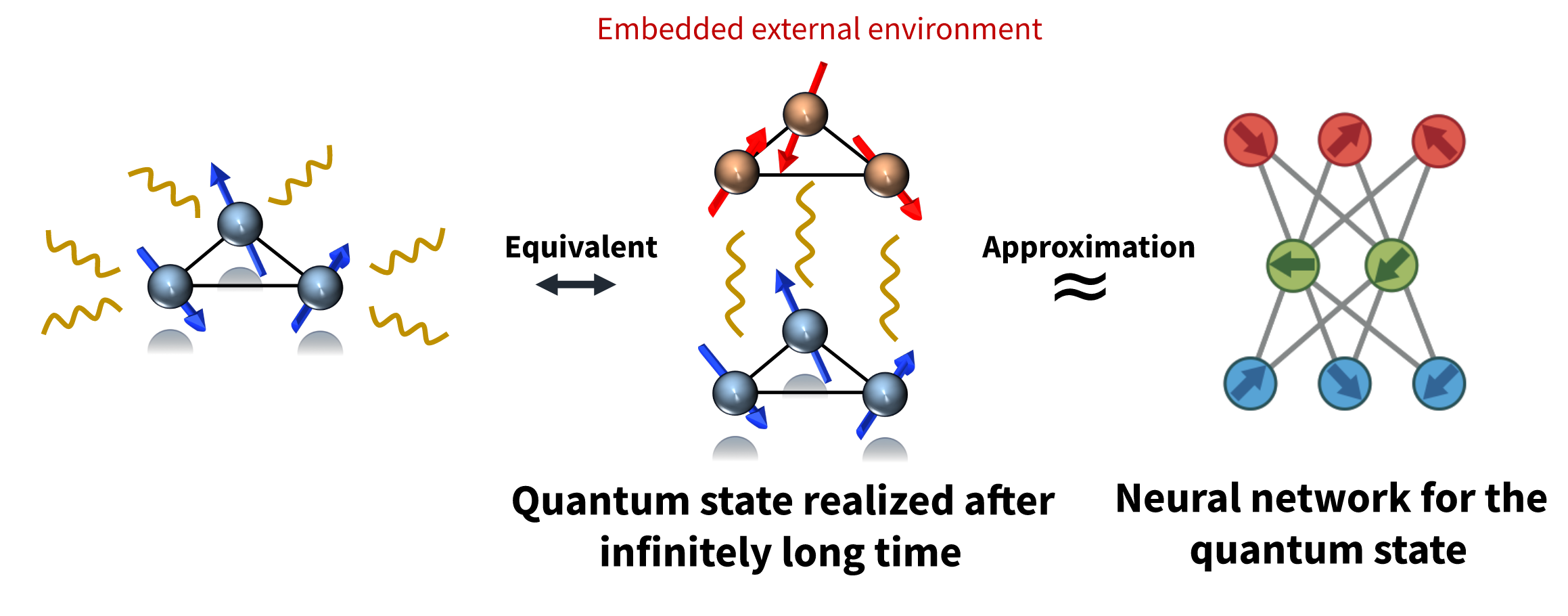
A diagram to represent how a neural network models a quantum system. Image: (c) 2019 Yoshioka et al.
Neural networks are sophisticated computer models. Traditional computer models can be fast at processing vast but simple data, but can be rather inflexible. The power of neural networks lies in the fact they can more easily grasp patterns in sets of data that traditional programs or even humans cannot see. This ability makes them much better at extracting the essence of natural phenomena like quantum physics so they can streamline things like simulations.
Yoshioka and his colleague Ryusuke Hamazaki have applied neural networks to real-world data about complex quantum systems. The neural networks spotted patterns in the vast mathematical data and were able to reduce the amount of time needed to run an effective simulation. Neural networks have been used to model isolated systems, but this is a first for quantum systems which interact with their environments.
“The process is complex, but broadly we take a random neural network as a ‘guess’ to represent the state of the quantum system,” said Hamazaki. “This state will be far from the expected real ‘answer.’ But through training the network by tweaking parameters over many iterations, the network produces ‘answers’ which correspond with expected laws of physics.”
Now that they’ve done it, what can be done with it?
There are two areas in particular that could benefit from these kinds of simulations. Semiconductor manufacturers create microchips made from tiny transistors. Over the years, these have been made so small that quantum mechanical effects now affect their behavior. The researchers’ simulations could be used to model ever-smaller transistors and possibly even transistors made from single atoms.
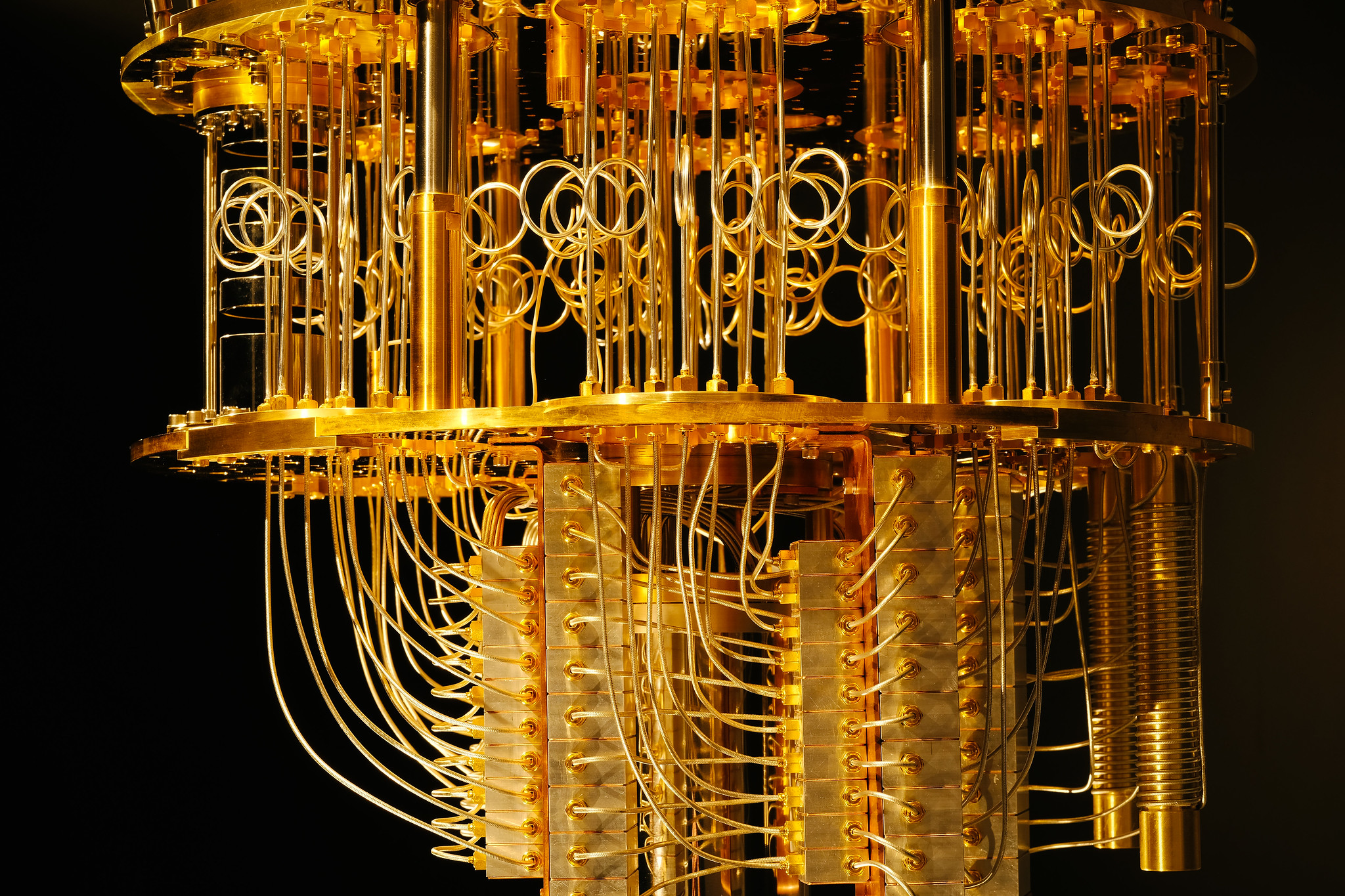
Building larger-scale quantum computers can benefit from this research. Image: Flickr/IBM Research CC BY-ND 2.0
The second area is research into building quantum computers, machines that could themselves be used to run highly detailed simulations, but that could also revolutionize privacy and security. Current investigations in this area focus on how to isolate sensitive components from the external environment; hence, why the researchers’ simulations would be so useful.
“It’s great to create something useful. Our research field, the intersection of machine learning and quantum physics, is so young that almost any idea is new and has potential use,” continued Yoshioka. “I’m a physicist so computer science is a new world for me. The ideas we work on are exciting because we feel they will contribute to the advancement of science.”
“What I found profound was the unexpectedly high performance of the neural networks,” concluded Hamazaki. “In computer science, neural networks are tools to generalize data, whereas in quantum physics, the goal is to produce specialized and accurate representations. It is very exciting that a single tool can achieve both.”
Papers
Nobuyuki Yoshioka and Ryusuke Hamazaki, "Constructing neural stationary states for open quantum many-body systems," Physical Review B 99, 214306: June 28, 2019, doi:10.1103/PhysRevB.99.214306.
Link (Publication)