Transfer Machine Learning
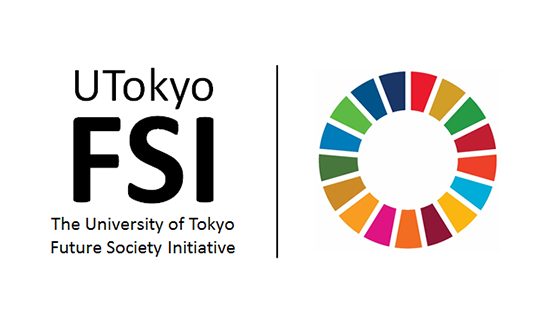
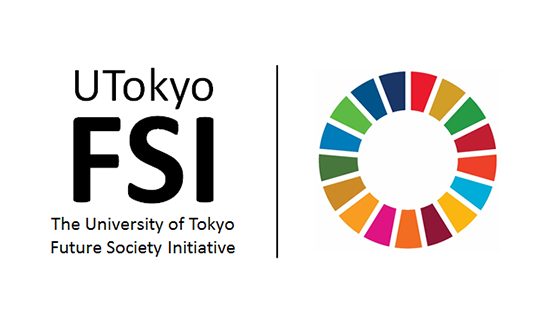
- 1.2 Data science
- 1.3 Artificial intelligence fundamentals
- 3.2 Mathematical and physical sciences
- 3.8 Informatics
Masashi Sugiyama
Graduate School of Frontier Sciences
Professor
Standard statistical machine learning methods assume that data used for training a predictor follow the same probability distribution as data to be predicted in the future test phase. This is a fundamental assumption that enables a predictor learned on training data to generalize to test data in the future. However, this basic assumption has frequently been violated in recent applications of machine learning. To cope with this problem, we are developing transfer learning methods for effectively reusing data used in similar tasks. In particular, we are exploring an approach based on importance weighting.
Related links
Research collaborators
RIKEN Center for Advanced Intelligence Project
Related publications
- Sugiyama, M. & Kawanabe, M. Machine Learning in Non-Stationary Environments: Introduction to Covariate Shift Adaptation, MIT Press, 2012. https://mitpress.mit.edu/books/machine-learning-non-stationary-environments
- Fang, T., Lu, N., Niu, G., & Sugiyama, M. Rethinking importance weighting for deep learning under distribution shift. In Advances in Neural Information Processing Systems 33, pp. 11996-12007, 2020. https://proceedings.neurips.cc/paper/2020/hash/8b9e7ab295e87570551db122a04c6f7c-Abstract.html
- Zhang, T., Yamane, I., Lu, N., & Sugiyama, M. A one-step approach to covariate shift adaptation. SN Computer Science, vol. 2, no. 319, 12 pages, 2021. https://link.springer.com/article/10.1007/s42979-021-00678-6
SDGs
Contact
- Masashi Sugiyama
- Email: sugi[at]k.u-tokyo.ac.jp
※[at]=@