Development of Machine-Learning for Interatomic Potential Models
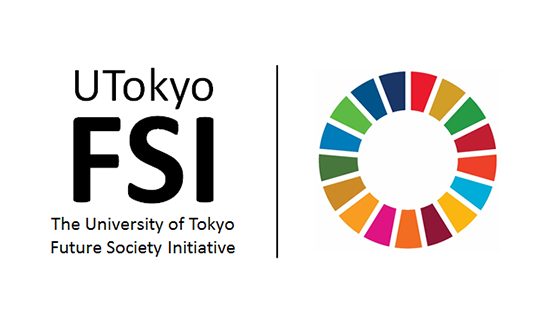
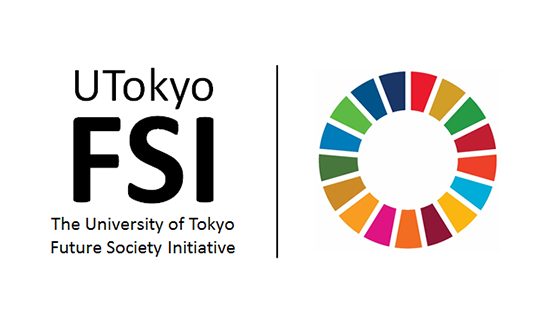
- 1.3 Statistics, Machine Learning, Data Assimilation, Algorithms, Mathematical Foundation, Data Mining
- 2.1 Material (Material Informatics, Remote Experiment, Laboratory Automation, etc.)
Satoshi Watanabe
Graduate School of Engineering
Professor
In this project, interatomic potential models enabling high accuracy and low computational load simulations of atomic structures of materials and their atomic dynamics are developed using first-principles calculation data and machine learning techniques.
Related links
Research collaborators
・Institute for Molecular Science
・National Institute of Advanced Industrial Science and
Technology
・Seoul National University, Korea
・National Institute of Advanced Industrial Science and
Technology
・Seoul National University, Korea
Related publications
Satoshi Watanabe, Wenwen Li, Wonseok Jeong, Dongheon Lee, Koji Shimizu, Emi Mimanitani, Yasunobu Ando, and Seungwu Han, “High-dimensional neural network atomic potentials for examining energy materials: some recent simulations,” Journal of Physics: Energy Vol. 3, No. 1, 012003, Jan. 2021.
SDGs
Contact
- Satoshi Watanabe, Graduate School of Engineering
- ex. 27135
- Tel: +81-3-5841-7135
- Email: watanabe[at]cello.t.u-tokyo.ac.jp
※[at]=@