Informatics for materials characterization
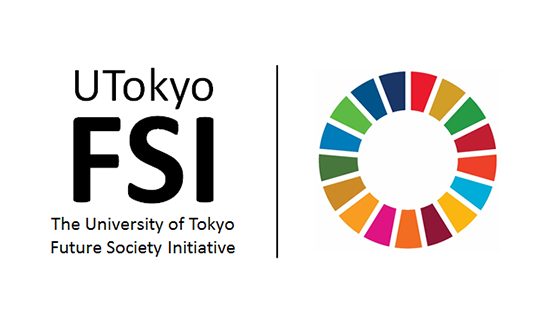
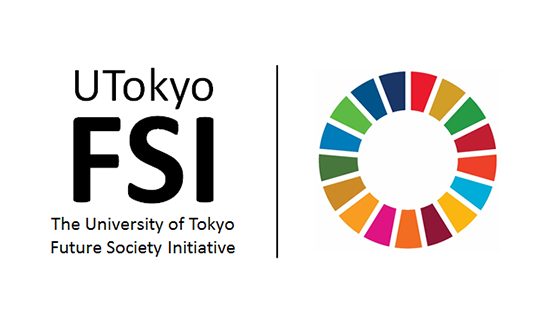
- 1.1 Data Processing Infrastructure (Cloud, Large-Scale Data Processing Systems, Machine Learning Frameworks, Databases, Data Structure)
- 2.1 Material (Material Informatics, Remote Experiment, Laboratory Automation, etc.)
- 2.2 Manufacturing Science and Engineering (Data-Driven Simulation, Structural Analysis, Fluid Analysis, Skills Transfer, Connected Industry)
- 2.3 Enviroment and Energy (Energy System, Renewable Energy, Ecosystem, Material Cycle, Life Cycle)
Teruyasu Mizoguchi
Institute of Industrial Science
Professor
Electron energy loss spectroscopy (EELS) and X-ray absorption spectroscopy (XAFS) are powerful material characterization techniques widely used to develop catalysts, batteries, and semiconductors. In this study, we developed a new method for material characterization using machine learning. The developed method was successful in extracting unknown information from the spectral data.
Related links
Research collaborators
・National Institute of Advanced Industrial Science and Technology (AIST)
・National Institute for Materials Science (NIMS)
・Kyoto University
・Hirosaki University
・National Institute for Materials Science (NIMS)
・Kyoto University
・Hirosaki University
Related publications
"Learning excited states from ground states by using an artificial neural network"
S. Kiyohara, M. Tsubaki, and T. Mizoguchi
npj Comp. Mater., 6 (2020) 68-1-6. DOI:10.1038/s41524-020-0336-3.
"Machine learning applications for ELNES/XANES"
T. Mizoguchi and S. Kiyohara
Microscopy, 69 (2020) 92-109. DOI:10.1093/jmicro/dfz109
"Quantitative estimation of properties from core-loss spectrum via neural network"
S. Kiyohara, M. Tsubaki, Kunyen Liao, and T. Mizoguchi
J. Phys.: Materials, 2 (2019) 024003-1-9.
"Data-driven approach for the prediction and interpretation of core-electron loss spectroscopy"
K. Kiyohara, T. Miyata, K. Tsuda, and T. Mizoguchi
Scientific Reports, 8 (2018) 13548-1-12.
S. Kiyohara, M. Tsubaki, and T. Mizoguchi
npj Comp. Mater., 6 (2020) 68-1-6. DOI:10.1038/s41524-020-0336-3.
"Machine learning applications for ELNES/XANES"
T. Mizoguchi and S. Kiyohara
Microscopy, 69 (2020) 92-109. DOI:10.1093/jmicro/dfz109
"Quantitative estimation of properties from core-loss spectrum via neural network"
S. Kiyohara, M. Tsubaki, Kunyen Liao, and T. Mizoguchi
J. Phys.: Materials, 2 (2019) 024003-1-9.
"Data-driven approach for the prediction and interpretation of core-electron loss spectroscopy"
K. Kiyohara, T. Miyata, K. Tsuda, and T. Mizoguchi
Scientific Reports, 8 (2018) 13548-1-12.
SDGs
Contact
- Teruyasu Mizoguchi
- Email: teru[at]iis.u-tokyo.ac.jp
※[at]=@