Weakly Supervised Machine Learning
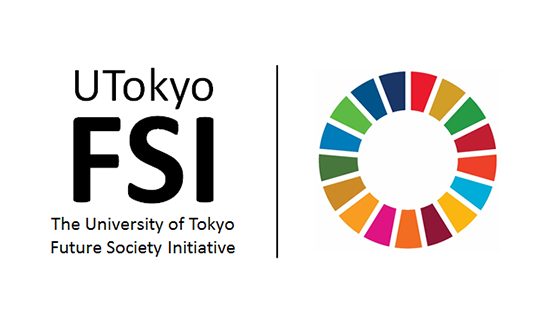
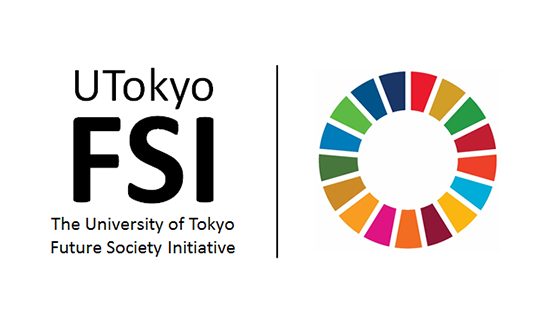
- 1.2 Data science
- 1.3 Artificial intelligence fundamentals
- 3.2 Mathematical and physical sciences
- 3.8 Informatics
Masashi Sugiyama
Graduate School of Frontier Sciences
Professor
In modern machine learning methods, predictors are trained using a large amount of fully annotated data. However, due to high labeling costs or privacy concerns, it is often difficult to obtain such full supervision. To solve this problem, we are developing a general framework for training a classifier under weak supervision. Our framework includes various weakly supervised learning problems, and we are exploring a systematic solution based on empirical risk minimization. This framework can also be combined with deep learning, enabling high generalization capability.
Related links
Research collaborators
RIKEN Center for Advanced Intelligence Project
Related publications
- du Plessis, M. C., Niu, G., & Sugiyama, M. Analysis of learning from positive and unlabeled data. NeurIPS2014, pp. 703-711, 2014. https://papers.nips.cc/paper/2014/hash/35051070e572e47d2c26c241ab88307f-Abstract.html
- Lu, N., Zhang, T., Niu, G., & Sugiyama, M. Mitigating overfitting in supervised classification from two unlabeled datasets: A consistent risk correction approach. AISTATS2020, pp. 1115-1125, 2020. https://proceedings.mlr.press/v108/lu20c.html
- Sugiyama, M., Bao, H., Ishida, T., Lu, N., Sakai, T., & Niu, G. Machine Learning from Weak Supervision: An Empirical Risk Minimization Approach, The MIT Press, to appear.
SDGs
Contact
- Masashi Sugiyama
- Email: sugi[at]k.u-tokyo.ac.jp
※[at]=@